Today we are at the moment of the emergence of the generative artificial intelligence (AI) rudiments of the future technological stack. Every year, hundreds of new startups flock to the market to develop learning models, build AI-based applications, and tools to interact with it, and build the infrastructure to support the work of it all.
Often, new technological trends become “bubbles” long before they really become popular in the market. But the boom in generative AI is accompanied by real advances in real markets and real nourishment from real companies. Models like Stable Diffusion or ChatGPT set historic user growth records, and several AI-powered apps have achieved annual revenue of $100 million less than a year after launch. And it’s time to recognize that in some tasks, artificial intelligence already surpasses people by several orders of magnitude. The question is: who makes the main profit from this and who will own it all in the future?
AI is the next promising business
Over the past year, we have met with dozens of founders and executives in startups in this field, as well as large companies that deal directly with generative AI. And we’ve noticed that most of the revenue from that comes from infrastructure providers. At the same time, companies that deal directly with applications are very quickly increasing their revenue, but at the same time, they have to deal with user retention. But most suppliers of AI models, although responsible for the very existence of this market, have not yet achieved great commercial success.
In other words, the companies that create the most value, i.e. learning generative AI models and applying them to new applications, haven’t captured much of the market when it comes to revenue. And it is still difficult to predict what will happen next. Let’s try to disassemble the stack of generative AI and understand which parts of it will really be in demand and subject to development, and who are the likely beneficiaries of this process.
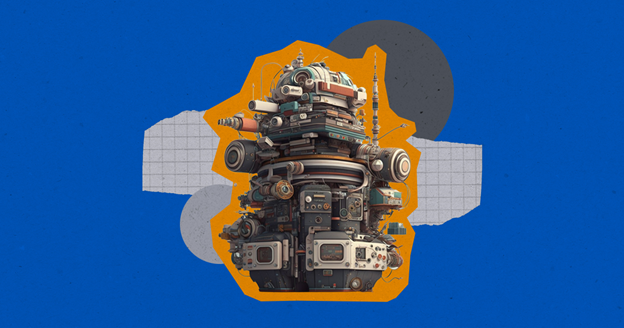
High-Level Technology Stack: Infrastructure, Models, and Applications
To understand how the market for generative AI is formed, we first need to determine what the stack looks like today. We see it like this:
The stack can be divided into three layers:
1. Applications that integrate generative AI models into a user-centric product, either by running their own model pipelines (“end-to-end applications”) or relying on a third-party API.
2. Models that “power” AI products, available either as proprietary APIs or as slices from open source solutions (which in turn also require hosting somewhere)
3. Infrastructure providers (i.e., cloud platforms and hardware manufacturers) that provide resources to train ai generative models and deliver results from working with them.
The first wave of applications based on generative AI shows tremendous growth, but struggles with retention and differentiation
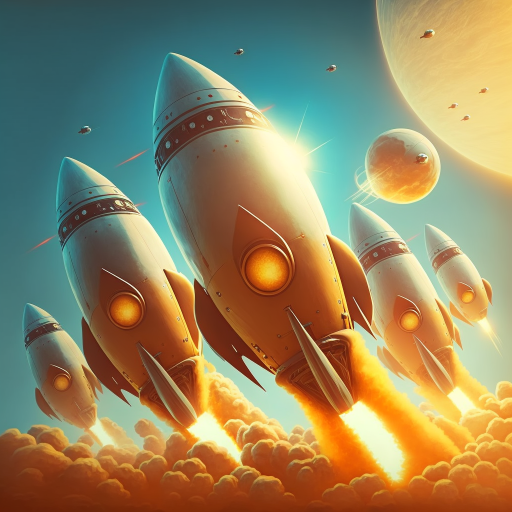
Previously (in previous technology cycles), it was believed that in order to create a large, independent company, you must “own” the end user. It does not matter whether these are individual consumers or buyers of the B2B segment. It would be tempting for ordinary users to assume that the largest companies in the field of generative AI will invest mainly in applications for end users, and not go to the market of “business big”, but so far this issue is unclear.
Yes, the growth of AI-powered generative applications has been staggering, driven by sheer novelty and a plethora of use cases. But we can definitely outline three categories of products whose annual revenues have already exceeded $ 100 million – these are image generation, copywriting and coding.
However, growth alone is not enough to create software companies that have been stable for a long time. It is critical that growth is also profitable, that is, when users and customers both generate profits immediately from the moment of their registration (high gross profit) and remain for a long time (high retention). In the absence of strong technical differentiation, B2B and B2C applications add long-term value to customers by increasing customer reach, storing intermediate information, or creating increasingly complex workflows.
Although we are now seeing a stampede at the “entrance to the marketing funnel”, but can current customer acquisition strategies become scalable? You can observe that the effectiveness of paid subscriptions and customer retention figures are beginning to decline. Many applications are at risk, as they often rely on similar basic AI models and do not contain any features that competitors would find difficult to duplicate.
It’s not yet clear whether selling apps to end users is the only or even the best way to build a sustainable business based on generative AI. In theory, the margin should increase as competition and the effectiveness of the language models used to increase. Retention should also increase as “AI tourists” leave the market (we are talking about a comparison with tourists who come only for a short period of time and permanent residents, translator’s note). We think that vertically integrated applications have an advantage in differentiation, but only time will tell.
Companies engaged in applications based on generative AI are now asking questions:
- Whether to do vertical integration (“model + application”). Using third-party AI model services allows application developers to quickly iterate with a small team and change model providers as technology evolves. On the other hand, some developers claim that their product is just a model and that only training from scratch and constant retraining based on proprietary data is the only protection against the creation of clones. But this requires a much greater investment in the project and limits the speed of the product team.
- Focus on features versus apps. Products based on generative AI come in several different forms: desktop apps, mobile apps, Figma/Photoshop plugins, Chrome extensions, and even Discord bots (hello Mindjourney). It’s easy to integrate AI-based products into what users are already working with, as the user interface is often just a text box. Which products do you think will become standalone companies and which will be absorbed by incumbent companies like Microsoft or Google already implementing AI in their product lines?
- Control through the HYIP cycle. It’s unclear whether user churn is only inherent in certain versions of products based on generative AI, or are they artifacts of an emerging market. Or will the surge of interest in generative AI subside as the hype on them subsides? These issues have important implications for application development companies, including when to “press the gas pedal” when fundraising; how aggressively to invest in attracting customers; what priorities should be set for different user segments, and when to declare the suitability of the product for the market.
Model vendors have invented generative AI, but have not had much success in commercializing them.
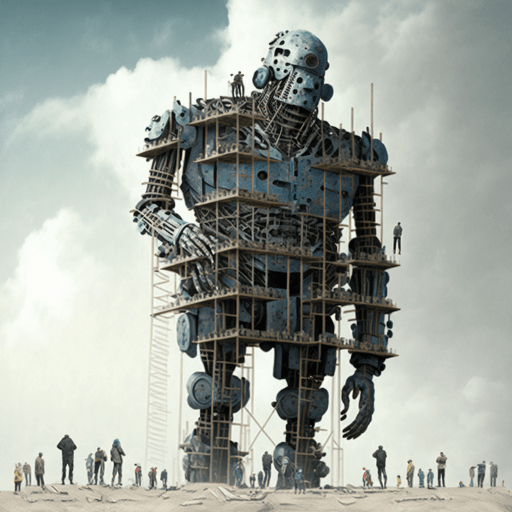
What we now call generative AI wouldn’t exist without the brilliant research and engineering work done by Google, OpenAI, and Stability. With new model architectures and a heroic effort to scale learning flows, we are all benefiting from the mind-blowing capabilities of today’s large language models (LLMs) and image generation models.
However, the income of the modelers themselves is still relatively small compared to the size of their use and the hype around them. Stable Diffusion demonstrates the explosive growth of the community, supported by an ecosystem of user interfaces, online service and fine-tuning techniques. But Stability gives away “slices” of its developments for free, putting it the main principle of its business. In natural language models, OpenAI dominates with GPT-3/3.5 and ChatGPT. But there are still relatively few killer applications built on OpenAI solutions, and the company itself has already been forced to reduce the prices of its services (and began the process of monetizing the same ChatGPT, translator’s note).
Yes, it may just be a temporary phenomenon. Stability is a young company that has not yet focused on monetization. OpenAI could become big business, taking a significant chunk of revenue in all natural language processing (NLP) categories as more killer apps are built, especially if they are successfully integrated into a multitude of Microsoft products. That is, the income from the models will be commensurate with the level of their use.
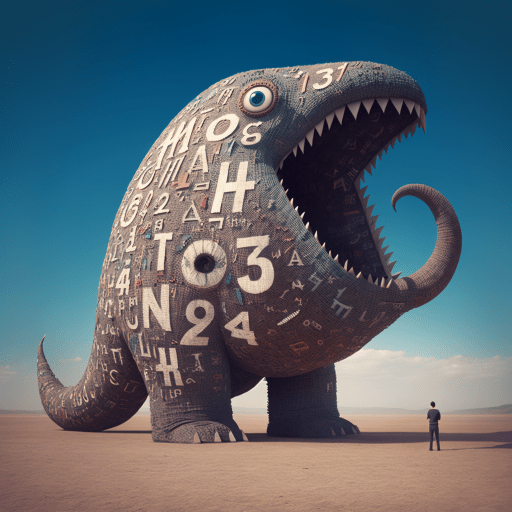
But there are also balancing forces – these are models released with open source. They can be placed by anyone, anywhere, including third-party companies that do not incur the costs associated with their large-scale training (and this is often tens or even hundreds of millions of dollars of costs). Therefore, it is unclear whether closed-source models will be able to maintain their advantage indefinitely. For example, LLMs built by Anthropic, Cohere, and Character.ai are already approaching the performance level of similar OpenAI models, and at the same time, they are all trained on similar data sets (that is, from the Internet). The Stable Diffusion example shows that if open-source models achieve a sufficient level of performance and community support, then proprietary alternatives can be difficult to compete with.
Perhaps the most obvious takeaway for model providers is that it’s not the models themselves that need to be commercialized, but their hosting. The demand for proprietary APIs (from the same OpenAI) is growing rapidly among application manufacturers. Hosting services for open source models (e.g., Hugging Face or Replicate) are becoming useful hubs for easy sharing and integration of models, and even building bridges between model makers and their consumers.
But model suppliers also face a number of big questions:
- From elite to public. There is a widespread belief that AI models will converge in performance over time. Talking to app developers, it’s clear that this hasn’t happened yet and we still have leaders in both text and graphics models. But their benefits are often based not on unique model architectures, but on access to funding, proprietary data, and a general shortage of AI talent. But how long will this elitism last?
- The risk of being out of a job. Relying on model providers is a great way for companies that are developing applications at the initial stage and even for building a business. But at the same time, these companies have the incentive to create and/or place their own models as soon as they reach certain sizes for customers and revenues. Many model vendors are in a position where most of their revenue is made up of only a few applications. What happens when these customers decide they can develop their own AI?
- Is money that important? The promise of generative AI is so great, and also potentially so harmful, that many model providers are forced to pose as public organizations, laying the public good as part of their mission to calm public opinion. Although this does not prevent them from receiving income from models. But the debate about whether the actions of model suppliers are selfless and whether they do not want to capture market segments (especially human labor) in this way do not subside.
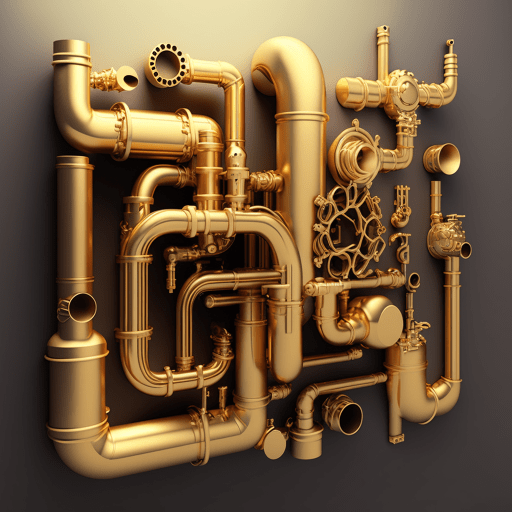
Infrastructure providers are catching up everywhere and reaping the benefits
Almost everything in generative AI at some point passes through a cloud-based GPU (or tensor processor – TPU). Whether it’s model providers, research labs, AI hosting companies, or all together, FLOPS are the lifeblood of generative AI. For the first time in a very long time, progress in revolutionary computing technology is largely related directly to computing.
As a result, most of the money in the generative AI market ends up going to infrastructure companies. We estimate that app companies spend about 20-40% of their revenue simply on displaying information and fine-tuning for each customer. Typically, this amount is paid either directly to cloud providers for compute instances or to third-party model providers, who in turn spend about half of their revenue on cloud infrastructure. Thus, it is reasonable to assume that at least 10-20% of the total revenue in generative AI today goes to cloud providers.
In addition, startups training their own models have raised billions of dollars in venture capital, and much of which tends to also be spent on cloud providers (sometimes up to 80–90% in the early rounds). Many public tech companies spend hundreds of millions of dollars a year training models. At the same time, they are invested either in external cloud providers or directly in equipment manufacturers.
And this is really “big money”, especially for the nascent market. Most of the funds are spent on the clouds of the “big three”: Amazon Web Services (AWS), Google Cloud Platform (GCP), and Microsoft Azure. These cloud providers have collectively invested nearly $100 billion a year in capital expenditures to secure the most complete, reliable, and cost-competitive platforms.
At the same time, startups such as Coreweave and Lambda Labs were able to wedge themselves into this market with solutions aimed specifically at large modelers. They compete on cost, availability, and personalized support and provide a more granular abstraction of resources (i.e., containers), while “big clouds” only offer VM instances due to the limitations of GPU virtualization.
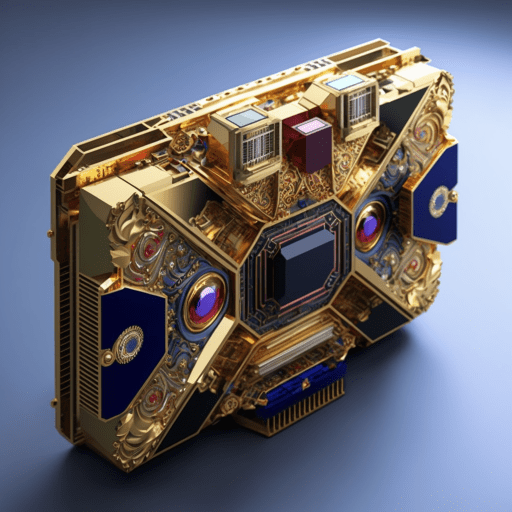
The gray cardinal and winner in terms of hardware for generative AI is Nvidia. Its revenue from GPUs for data centers in the third quarter of fiscal 2023 amounted to $ 3.8 billion, and a significant part was brought by the scenarios of using generative AI. The company has dug a robust “moat” around this business through decades of investment in GPU architecture, a robust software ecosystem, and mass adoption in the academic community. Nvidia GPUs are cited in research papers 90 times more often than the best AI chip startups combined.
There are other hardware options, including Google tensor processors, AMD Instinct GPUs, AWS Inferentia and Trainium chips. Also on the market are AI accelerators from startups such as Cerebras, Sambanova, and Graphcore. Intel, alas late to the distribution of “elephant AI“, enters the market with its high-end Habana chips and Ponte Vecchio graphics processors. But so far, few of these new chips have taken a significant market share. The two exceptions are Google, whose TPUs have gained popularity in the Stable Diffusion community and with some major cloud customers and, oddly enough, TSMC, which most likely makes all the chips listed here, including Nvidia GPUs (Intel uses both its own factories and TSMC’s power to make its chips).
In other words, infrastructure is the profitable, durable, and seemingly most secure layer in the generative AI stack. But even here certain questions arise:
- Workloads, not data retention. Nvidia GPUs are the same wherever you rent them. Most AI workflows do not require long-term data retention in the sense that the output of the model does not require attaching huge databases to it (except for the scales of the model themselves). This means that the AI workload can be easily transferred from one cloud to another what prevents the customer from switching to the cheapest cloud service provider in this case?
- The end of the shortage of chips. The high prices of cloud providers and Nvidia itself are now supported by the meager supply of the most coveted GPUs. But when this supply constraint is addressed by increased production and/or the introduction of new hardware platforms, how will it affect cloud providers and Nvidia itself?
- Can competitors break through this? We are confident that vertical clouds will take market share away from the Big 3 with more specialized offerings. And so far, the competitor in the field of infrastructure for AI has been successful only due to the fact that the rest of us had everything in the same way since for the same Nvidia, existing cloud providers are both the largest customers and new competitors. The question is, will “not be like everyone else” be enough to overcome the massive advantages of the Big 3 that have begun to suspect something?
So… and who will have all the profits?
We don’t know for sure, but we can assume that there’s no way today to “dig a ditch” around their generative AI business to protect against the onslaught of competitors: apps lack a differentiating feature for their product because they use similar models; Models face unclear long-term differentiation as they learn from similar datasets and similar architectures. cloud providers lack deep technical differentiation because they use the same GPUs, and even chipmakers produce them in the same factories.
Of course, you can crush competitors at scale (“I have or can raise more money than you!”), supply chains (“I have GPUs, you don’t have them!”), ecosystem (“Everyone is already using my software!”), algorithms (“We’re smarter than you!”), marketing (“I already have a sales team and more customers than you!”) and even the sample size of the data (“I’ve processed more internet than you!”). But none of the methods of protection is durable in the long run. And it’s too early to say whether there is a “crowd effect” at any level of the stack and whether there will be a single winner in the market.
That’s weird. But that’s good news for us. The potential size of this market is difficult to understand. It lies somewhere between all the software and all the human effort, so we expect even more players to arrive and healthy competition at all levels of the stack. We also expect both horizontal and vertical companies to succeed, with the best approach dictated by end markets and end users.
0 Comments