SoundStorm
Parallel Audio Generation
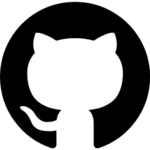
SoundStorm is a model for efficient, non-autoregressive audio generation. It takes the semantic tokens of AudioLM as input and uses bidirectional attention and confidence-based parallel decoding to generate the tokens of a neural audio codec. SoundStorm can produce high-quality audio faster and more consistently than the autoregressive approach of AudioLM. It can also synthesize natural dialogue segments from a transcript with speaker turns and voice prompts.
Install
$ pip install soundstorm-pytorch
Usage
import torch
from soundstorm_pytorch import SoundStorm, ConformerWrapper
conformer = ConformerWrapper(
codebook_size = 1024,
num_quantizers = 4,
conformer = dict(
dim = 512,
depth = 2
),
)
model = SoundStorm(
conformer,
steps = 18, # 18 steps, as in original maskgit paper
schedule = 'cosine' # currently the best schedule is cosine
)
# get your pre-encoded codebook ids from the soundstream from a lot of raw audio
codes = torch.randint(0, 1024, (2, 1024))
# do the below in a loop for a ton of data
loss, _ = model(codes)
loss.backward()
# model can now generate in 18 steps. ~2 seconds sounds reasonable
generated = model.generate(1024, batch_size = 2) # (2, 1024)
To use raw audio as input, you have to feed your pre-trained SoundStream
model to SoundStorm
. You can train your own SoundStream
model with audiolm-pytorch.
import torch
from soundstorm_pytorch import SoundStorm, ConformerWrapper, Conformer, SoundStream
conformer = ConformerWrapper(
codebook_size = 1024,
num_quantizers = 4,
conformer = dict(
dim = 512,
depth = 2
),
)
soundstream = SoundStream(
codebook_size = 1024,
rq_num_quantizers = 4,
attn_window_size = 128,
attn_depth = 2
)
model = SoundStorm(
conformer,
soundstream = soundstream # pass in the soundstream
)
# find as much audio you'd like the model to learn
audio = torch.randn(2, 10080)
# course it through the model and take a gazillion tiny steps
loss, _ = model(audio)
loss.backward()
# and now you can generate state-of-the-art speech
generated_audio = model.generate(seconds = 30, batch_size = 2) # generate 30 seconds of audio (it will calculate the length in seconds based off the sampling frequency and cumulative downsamples in the soundstream passed in above)
0 Comments