ChatGLM2-6B
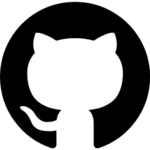
ChatGLM 2-6B is an open-source Chinese-English bilingual dialogue model that builds on the original ChatGLM-6B. It inherits the advantages of the first-generation model, such as fluent conversation and easy deployment.
Model | Average | STEM | Social Sciences | Humanities | Others |
---|---|---|---|---|---|
ChatGLM-6B | 40.63 | 33.89 | 44.84 | 39.02 | 45.71 |
ChatGLM2-6B (base) | 47.86 | 41.20 | 54.44 | 43.66 | 54.46 |
ChatGLM2-6B | 45.46 | 40.06 | 51.61 | 41.23 | 51.24 |
C-Eval
Model | Average | STEM | Social Sciences | Humanities | Others |
---|---|---|---|---|---|
ChatGLM-6B | 38.9 | 33.3 | 48.3 | 41.3 | 38.0 |
ChatGLM2-6B (base) | 51.7 | 48.6 | 60.5 | 51.3 | 49.8 |
ChatGLM2-6B | 50.1 | 46.4 | 60.4 | 50.6 | 46.9 |
GSM8K
Model | Accuracy | Accuracy (Chinese)* |
---|---|---|
ChatGLM-6B | 4.82 | 5.85 |
ChatGLM2-6B (base) | 32.37 | 28.95 |
ChatGLM2-6B | 28.05 | 20.45 |
BBH
Model | Accuracy |
---|---|
ChatGLM-6B | 18.73 |
ChatGLM2-6B (base) | 33.68 |
ChatGLM2-6B | 30.00 |
Environment installation
To get started, clone this repository:
git clone https://github.com/THUDM/ChatGLM2-6B
cd ChatGLM2-6B
To install the required libraries, run pip with the following command, specifying the recommended version or higher for optimal inference speed: pip install -r requirements.txt
transformers
4.30.2
torch
Load the model locally
To run the code, you need to download the model from Hugging Face Hub. This is a full model that the code will use automatically. If you have a slow or unstable internet connection, you might face issues or delays in downloading the model. A possible solution is to download the model to your local machine and then load it from there.transformers
To get a model from Hugging Face Hub, you need to set up Git LFS and run it.
git clone https://huggingface.co/THUDM/chatglm2-6b
A faster way to get models from Hugging Face Hub is to download only the model code, without the checkpoints.
GIT_LFS_SKIP_SMUDGE=1 git clone https://huggingface.co/THUDM/chatglm2-6b
To update the model parameter file, follow this link and save the file to your local directory, overwriting the existing one.chatglm2-6b
o use the model from your local folder, you need to change the code above with the local folder path where you saved the model.THUDM/chatglm2-6b
chatglm2-6b
The model implementation may change over time. To ensure compatibility with the model implementation invariant, you can increase the argument when you invoke. is the most recent version number, see Change Log for all versions.from_pretrained
revision="v1.0"
v1.0
Web Demo
First, install Gradio: then run the web_demo.py in the repository:pip install gradio
python web_demo.py
The output address of the program, which operates a Web server, can be opened in a browser for functionality.
@AdamBear deserves our gratitude for creating the web demo with Streamlit. To run it, you have to install these extra dependencies first:web_demo2.py
pip install streamlit streamlit-chat
Use this command to launch it:
streamlit run web_demo2.py
The web demo works better with Streamlit when the input prompt is longer.
Command line Demo
Run the cli_demo.py in the repository:
python cli_demo.py
The program will use the command line to communicate with the user. The user can type commands and press enter to get a response from the program. To reset the conversation history, the user can press enter without typing anything. To exit the program, the user can type terminate and press enter.clear
stop
API deployment
Before running the api.py file, make sure you have installed all the required packages from the repository:pip install fastapi uvicorn
python api.py
The POST method is used to call the deployment, which runs on port 8000 on-premises by default.
curl -X POST "http://127.0.0.1:8000" \
-H 'Content-Type: application/json' \
-d '{"prompt": "你好", "history": []}'
The resulting return value is
{
"response":"你好👋!我是人工智能助手 ChatGLM2-6B,很高兴见到你,欢迎问我任何问题。",
"history":[["你好","你好👋!我是人工智能助手 ChatGLM2-6B,很高兴见到你,欢迎问我任何问题。"]],
"status":200,
"time":"2023-03-23 21:38:40"
}
We appreciate @hiyouga’s contribution to the OpenAI-formatted streaming API deployment, which enables any ChatGPT-based application, such as ChatGPT-Next-Web. to use it as a backend. To deploy, simply run openai_api.py in your repository:
python openai_api.py
This is the sample code for making an API call:
import openai
if __name__ == "__main__":
openai.api_base = "http://localhost:8000/v1"
openai.api_key = "none"
for chunk in openai.ChatCompletion.create(
model="chatglm2-6b",
messages=[
{"role": "user", "content": "你好"}
],
stream=True
):
if hasattr(chunk.choices[0].delta, "content"):
print(chunk.choices[0].delta.content, end="", flush=True)
Low-cost deployment
Model quantization
To reduce the video memory usage, you can load the model with quantization instead of FP16 precision. The following code shows how to do this. The model needs about 13GB of video memory with FP16 precision.
# 按需修改,目前只支持 4/8 bit 量化
model = AutoModel.from_pretrained("THUDM/chatglm2-6b", trust_remote_code=True).quantize(8).cuda()
Reducing the model to 4 bits affects its performance, but ChatGLM2-6B can generate natural and smooth texts even with this quantization.
A quantized model can help you save memory. To use it, just load it directly:
model = AutoModel.from_pretrained("THUDM/chatglm2-6b-int4",trust_remote_code=True).cuda()
CPU deployment
You can perform inference on the CPU if you lack GPU hardware, but it will take longer. Follow these steps to use it (you need about 32GB RAM).
model = AutoModel.from_pretrained("THUDM/chatglm2-6b", trust_remote_code=True).float()
A quantized model can reduce memory consumption when the available memory is insufficient.
model = AutoModel.from_pretrained("THUDM/chatglm2-6b-int4",trust_remote_code=True).float()
To use the quantized model on the CPU, you need to install it. These are usually pre-installed on Linux systems. For Windows, make sure to select when you install TDM-GCC. Refer to Q1 on MacOS.gcc
openmp
openmp
gcc
TDM-GCC 10.3.0
gcc 11.3.0
0 Comments