ARB
Advanced Reasoning Benchmark
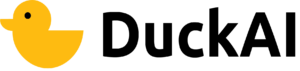
Duck AI ARB (Advanced Reasoning Benchmark) is a new dataset that contains complex reasoning tasks to measure the performance of LLMs on text understanding and domain-specific reasoning. It is more difficult than previous benchmarks, as it includes questions that require deeper knowledge of mathematics, physics, biology, chemistry, and law.
Math Symbolic
Math Proof-like
Physics Symbolic
Evaluation Results
Current large language models (LLMs) are mainly tested on text-only problems, without any multimodal tasks, using models such as ChatGPT, GPT 3.5, GPT-4, and Claude. The questions are different for each problem type, with specific instructions and reasoning steps; for multiple-choice questions, the model’s answer is checked against the right one, while numerical, symbolic, and proof-like problems need to extract and parse the model’s answer, which can be very complex and need mathematical tools and human grading. The study also tried two methods for grading based on models, including GPT-4‘s skill to grade two symbolic expressions for equivalence and a method based on a rubric, which showed good results, making it easier to evaluate more unstructured answers.
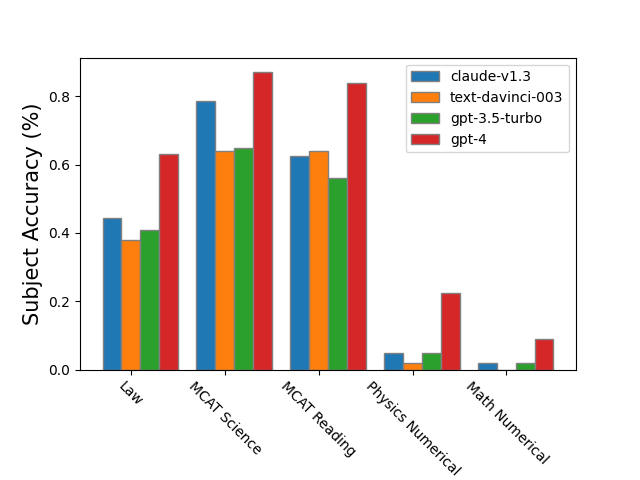
Model-based Rubric Evaluation
One challenge for evaluating language learning models (LLMs) that perform complex reasoning tasks is how to grade symbolic answers and check intermediate reasoning steps. The study suggests a method where the model produces and applies rubrics to assess solutions, based on reference solutions and examples of human-made rubrics. The evaluation showed that GPT-4 generates effective rubrics, covering key solution steps well but having difficulties with point allocation, surpassing its predecessor, GPT-3.5-turbo.
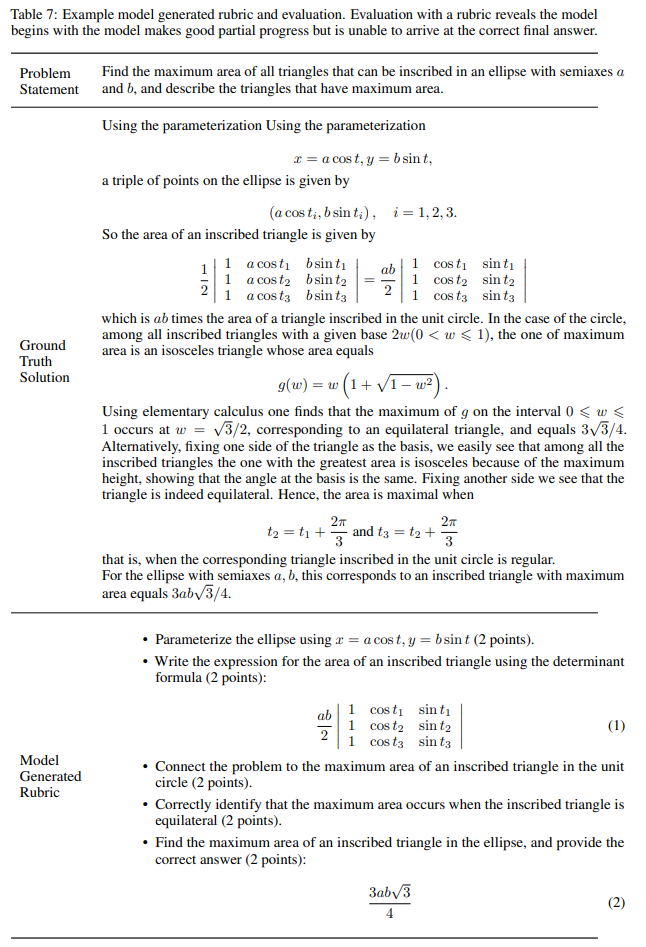
0 Comments