Audiocraft
Controllable Music Generation
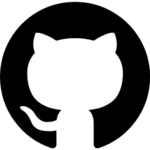
Audiocraft is a PyTorch library for audio generation research. It contains MusicGen, a controllable text-to-music model. MusicGen is a Transformer that generates 4 codebooks sampled at 50Hz. Unlike MusicLM, MusicGen generates all codebooks in 1 pass with a small delay, needing only 50 autoregressive steps/sec.
MusicGen is a model for music generation that Audiocraft provides the code and models. It is a single-stage auto-regressive Transformer model that uses a 32kHz EnCodec tokenizer with 4 codebooks sampled at 50 Hz. MusicGen doesn’t need a self-supervised semantic representation like MusicLM, and it generates all 4 codebooks at once. By adding a small delay between the codebooks, MusicGen can parallelize their prediction, and reduce the auto-regressive steps to 50 per second of audio.
Installation
To use Audiocraft, you need Python 3.9, PyTorch 2.0.0, and a GPU with at least 16 GB of memory (for the medium-sized model). You can install Audiocraft by running this command:
# Best to make sure you have torch installed first, in particular before installing xformers.
# Don't run this if you already have PyTorch installed.
pip install 'torch>=2.0'
# Then proceed to one of the following
pip install -U audiocraft # stable release
pip install -U git+https://git@github.com/facebookresearch/audiocraft#egg=audiocraft # bleeding edge
pip install -e . # or if you cloned the repo locally
Usage
At Audiocraft, various modes of engagement with MusicGen are available:
- To try MusicGen, you have two options: run the jupyter notebook at
demo.ipynb
on your own machine, or use the provided colab notebook. - To launch the gradio demo locally, run
python app.py
. - A demo is also available on theÂ
facebook/MusicGen
 HuggingFace Space . - Finally, apply a Gradio demo with a Colab GPU, follow the steps from @camenduru Colab.
API
Audiocraft offers a simple API and 4 ready-made models. The models are:
small
: 300M model, text to music only – 🤗 Hubmedium
: 1.5B model, text to music only – 🤗 Hubmelody
: 1.5B model, text to music and text+melody to music – 🤗 Hublarge
: 3.3B model, text to music only – 🤗 Hub
 The medium
 or melody
model gives the optimal balance of quality and computing. MusicGen requires a GPU to run locally. We suggest 16GB of memory, but you can generate short sequences or use the small
model with less memory.
Below is a brief demonstration of how to use the API.
import torchaudio
from audiocraft.models import MusicGen
from audiocraft.data.audio import audio_write
model = MusicGen.get_pretrained('melody')
model.set_generation_params(duration=8) # generate 8 seconds.
wav = model.generate_unconditional(4) # generates 4 unconditional audio samples
descriptions = ['happy rock', 'energetic EDM', 'sad jazz']
wav = model.generate(descriptions) # generates 3 samples.
melody, sr = torchaudio.load('./assets/bach.mp3')
# generates using the melody from the given audio and the provided descriptions.
wav = model.generate_with_chroma(descriptions, melody[None].expand(3, -1, -1), sr)
for idx, one_wav in enumerate(wav):
# Will save under {idx}.wav, with loudness normalization at -14 db LUFS.
audio_write(f'{idx}', one_wav.cpu(), model.sample_rate, strategy="loudness", loudness_compressor=True)
0 Comments